Data validation is an essential step in any data processing pipeline, as it ensures the integrity and accuracy of the data to be used across all subsequent processing steps. Great Expectations (GX) is an open-source framework that provides a flexible and efficient way to perform data validation, allowing data scientists and analysts to quickly identify and correct any issues with their data. In this article, we share our experience implementing Great Expectations for data validation in our Hadoop environment, and our take on its benefits and limitations.
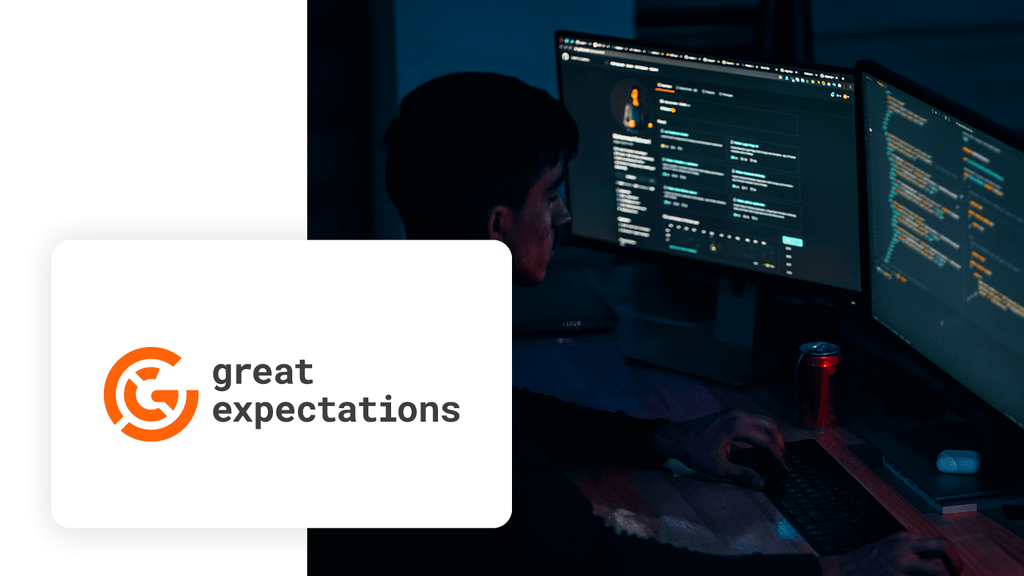
Follow us on